Easy Contact
253 Main Ave, Passaic NJ 07055Call 973-777-5656
info@advantagecomputers.com
Fax 973-777-5821
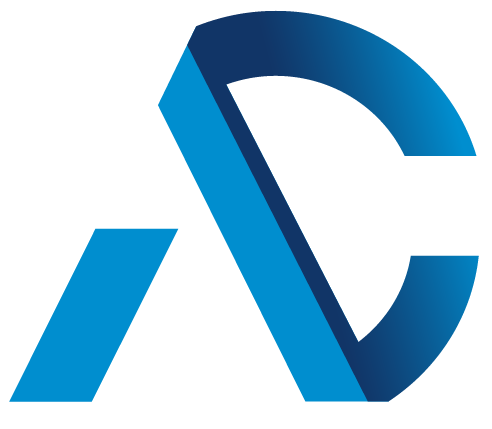
© 2025 ~ All Rights Reserved
Advantage Computer Solutions, Inc
Company
Services
Testimonials
Zack is amazing! I have gone to him with computer issues for the past few years now and he always finds a way to fix things and at a reasonable price. This time I went to Advantage Computer Solutions to find a new laptop. I needed help because like most of us I had no… Read more “Amazing!”
Cannot say enough good things about Zack Rahhal and his team. Professional, smart, sensitive to small biz budgets and a helluva good guy. Could not operate my small biz without them!
stars indeed. So reliable and helpful and kind and smart. We call Al and he is “on it” immediately and such a FABULOUS teacher, patient and terrific. So happy with Advantage Computer Solutions and Al and his AMAZINGLY WONDERFUL STAFF.
I’ve been a customer of the staff at Advantage for many years now. They have never let me down! Whatever my need, however big or small my problem, they have been unfailingly helpful, friendly and professional. Services are performed promptly and effectively, and they are very fair with pricing, too. I am lucky to have… Read more “Whatever my need, unfailingly helpful”
I’ve known the Advantage Team for years. They are the absolute best techs in the field, bar none. I couldn’t tell you how many tens thousands of dollars they saved us over the years; they can be trusted to never scam anyone even though they would do so very easily. The turnaround time is also… Read more “Best Kept Secret”
I had an excellent experience with Advantage. Aside from being extremely professional and pleasant generally, Zack was incredibly responsive and helpful, even before and after my appointment, and really resolved IT issues in my home office that had been plaguing me for years. I am so relieved to not have to think about this anymore!… Read more “Excellent Experience”
Simply The Best! Our company has been working with Advantage Computer Solutions for a few years, Zack and his Team are AWESOME! They are super reliable – whether it’s everyday maintenance or emergencies that may arise, The Advantage Team take care of us! Our team is grateful for their knowledgeable and professional services – a… Read more “Simply The Best!”
The engineering team at Advantage Computers is the best in the business. They are nothing short of technical wizards.
Al, Nasser and Zack have been keeping our operations going for over a decade, taking care of our regular upgrades and our emergency system problems. When we have an emergency, they make it their emergency. Its like having a cousin in the business.
In many cases, exceptional people do not receive recognition for their hard work and superior customer service. We do not want this to be one of those times. Zack Rahhal has been our hardware and technical consultant for our servers, Pc’s and other technical equipment since April 2004 and has provided valuable input and courteous service to… Read more “Exceptional People”
I became a customer about 6-7 months and I can say nothing but great things about this business. Zack takes care of me. I am an attorney and operate my own small firm. I have limited knowledge of computers. Zack is very patient in explaining things. He has offered practical and economical solutions to multiple… Read more “Highly Recommended”
THANK GOD for this local computer repair business who saved me hundreds, my hard drive was messed up, i called the company with warranty they said it would be $600, I went in they did a quick diagnostic, and based on his observations he gave me a step by step of the possible problems and… Read more “Life Savers”
I don’t have enough words to express my appreciation for Nassar and Paul, and the other members of Advantage Computer Solutions. I live in Bergen County and travel to Passaic County because of the trust I have in the competence and honesty of Advantage Computers. What a blessing to have such seasoned and caring professionals… Read more “I don’t have enough words to express my appreciation”
Advantage Computer Solutions is absolutely great. They show up, do what they say they are going to, complete the job without issues (my other computer companies had to keep coming back to fix things they “forgot” to do….) and are fairly priced. Zack is awesome, reliable, dependable, knowledgeable….everything you want in a computer solutions vendor.
Knowledgeable, Reliable, Reasonable Working with Advantage Computers since 1997 for both personal and business tech support has been a rewarding and enjoyable experience. Rewarding, in that the staff is very knowledgeable, approaching needs and issues in a very straightforward, common sense manner, resulting in timely solutions and resolutions. Enjoyable, these guys are really friendly (not… Read more “Knowledgeable, Reliable, Reasonable”
Excellent service! I am the administrator for a busy medical office which relies heavily on our computer system. We have used Advantage Computer Solutions for installation, set-up and for service. The response time is immediate and the staff is often able to provide help remotely. Very affordable and honest…. A++!!! Essex Surgical relies on Advantage… Read more “Excellent service!”
Advantage offers great advice and service I bought parts for my gaming pc online and they put it together in a day for a great price. They are very professional. I was very satisfied with their service. I am a newbie in terms of PC gaming so they gave me great advice on this new piece… Read more “Great Advice and Service”
Our company has been using the services of Advantage Computers since 2006. It was important to find a reliable company to provide us with the technical support both onsite and offsite. It was through a recommendation that we contacted Advantage to have them provide us with a quote to install a new server and update our… Read more “Great Service, Support and Sales”
Our company has been working with Advantage since the 1990’s and have been a loyal client ever since. Advantage does not make it very difficult to be loyal as they offer services from the most intricate and personalized to the global scale. Our company has grown beyond its doors of a local office to National… Read more “Extremely Professional and Passionate”
Advantage Computer Solutions has handled all of our computer and IT needs for the past 2 years. The staff is always professional and the service is always prompt. When your computers are down or not working properly is affects all aspects of your business, it is wonderful to have such a reliable team on our… Read more “Handles all our Office IT”
Since 1996 the Housing Authority of the City of Passaic has been a client of Advantage Computer Solutions. Our Agency has utilized their outstanding services and expertise to solve our technologic problems and growth over the past eighteen years. We would like to personally thank them for proposing cost effective solutions while reducing labor-intense tasks… Read more “Passaic Housing Authority”
“When the computer I use to run my photography business started acting erratically and kept shutting down, I was in a panic. I depend on that computer to deliver final products to my clients. Fortunately, I brought my HP into Advantage for repair and in one day I had my computer back. Not only did… Read more “They made sure EVERYTHING was working”
Voice assistants can be hacked with ultrasonic waves
With access to text messages and the ability to make fraudulent phone calls, attackers could wreak more damage than you’d think
The post Voice assistants can be hacked with ultrasonic waves appeared first on WeLiveSecurity
Brave comes out on top in browser privacy study
By contrast, two web browsers share identifiers that are tied to the device hardware and so persist even across fresh installs
The post Brave comes out on top in browser privacy study appeared first on WeLiveSecurity
5 reasons to consider a career in cybersecurity
From competitive salaries to ever-evolving job descriptions, there are myriad reasons why a cybersecurity career could be right for you
The post 5 reasons to consider a career in cybersecurity appeared first on WeLiveSecurity
FuzzBench: Fuzzer Benchmarking as a Service
Posted by Jonathan Metzman, Abhishek Arya, Google OSS-Fuzz Team and László Szekeres, Google Software Analysis Team
We are excited to launch FuzzBench, a fully automated, open source, free service for evaluating fuzzers. The goal of FuzzBench is to make it painless to rigorously evaluate fuzzing research and make fuzzing research easier for the community to adopt.
Fuzzing is an important bug finding technique. At Google, we’ve found tens of thousands of bugs (1, 2) with fuzzers like libFuzzer and AFL. There are numerous research papers that either improve upon these tools (e.g. MOpt-AFL, AFLFast, etc) or introduce new techniques (e.g. Driller, QSYM, etc) for bug finding. However, it is hard to know how well these new tools and techniques generalize on a large set of real world programs. Though research normally includes evaluations, these often have shortcomings—they don’t use a large and diverse set of real world benchmarks, use few trials, use short trials, or lack statistical tests to illustrate if findings are significant. This is understandable since full scale experiments can be prohibitively expensive for researchers. For example, a 24-hour, 10-trial, 10 fuzzer, 20 benchmark experiment would require 2,000 CPUs to complete in a day.
To help solve these issues the OSS-Fuzz team is launching FuzzBench, a fully automated, open source, free service. FuzzBench provides a framework for painlessly evaluating fuzzers in a reproducible way. To use FuzzBench, researchers can simply integrate a fuzzer and FuzzBench will run an experiment for 24 hours with many trials and real world benchmarks. Based on data from this experiment, FuzzBench will produce a report comparing the performance of the fuzzer to others and give insights into the strengths and weaknesses of each fuzzer. This should allow researchers to focus more of their time on perfecting techniques and less time setting up evaluations and dealing with existing fuzzers.
Integrating a fuzzer with FuzzBench is simple as most integrations are less than 50 lines of code (example). Once a fuzzer is integrated, it can fuzz almost all 250+ OSS-Fuzz projects out of the box. We have already integrated ten fuzzers, including AFL, LibFuzzer, Honggfuzz, and several academic projects such as QSYM and Eclipser.
Reports include statistical tests to give an idea how likely it is that performance differences between fuzzers are simply due to chance, as well as the raw data so researchers can do their own analysis. Performance is determined by the amount of covered program edges, though we plan on adding crashes as a performance metric. You can view a sample report here.
How to Participate
Our goal is to develop FuzzBench with community contributions and input so that it becomes the gold standard for fuzzer evaluation. We invite members of the fuzzing research community to contribute their fuzzers and techniques, even while they are in development. Better evaluations will lead to more adoption and greater impact for fuzzing research.
We also encourage contributions of better ideas and techniques for evaluating fuzzers. Though we have made some progress on this problem, we have not solved it and we need the community’s help in developing these best practices.
Please join us by contributing to the FuzzBench repo on GitHub.
RSA 2020 – Is your machine learning/quantum computer lying to you?
And how would you know if the algorithm was tampered with?
The post RSA 2020 – Is your machine learning/quantum computer lying to you? appeared first on WeLiveSecurity
Week in security with Tony Anscombe
ESET research uncovers a vulnerability in Wi-Fi chips – How to protect yourself against tax refund fraud – Clearview AI suffers a data breach
The post Week in security with Tony Anscombe appeared first on WeLiveSecurity
Firefox turns on DNS over HTTPS by default for US users
Users in other parts of the world also have the option to flip on DNS encryption
The post Firefox turns on DNS over HTTPS by default for US users appeared first on WeLiveSecurity
Cyberbullying: How is it different from face‑to‑face bullying?
The digital age has added a whole new dimension to hurtful behavior, and we look at some of the key features that set in-person and online bullying apart
The post Cyberbullying: How is it different from face‑to‑face bullying? appeared first on WeLiveSecurity
Helping Developers with Permission Requests
Posted by Sai Teja Peddinti, Nina Taft and Igor Bilogrevic from PDPO Applied Privacy Research, and Pauline Anthonysamy from Android Security and Privacy.
User trust is critical to the success of developers of every size. On the Google Play Store, we aim to help developers boost the trust of their users, by surfacing signals in the Developer Console about how to improve their privacy posture. Towards this aim, we surface a message to developers when we think their app is asking for permission that is likely unnecessary.
This is important because numerous studies have shown that user trust can be affected when the purpose of a permission is not clear.1 In addition, research has shown that when users are given a choice between similar apps, and one of them requests fewer permissions than the other, they choose the app with fewer permissions.2
Determining whether or not a permission request is necessary can be challenging. Android developers request permissions in their apps for many reasons – some related to core functionality, and others related to personalization, testing, advertising, and other factors. To do this, we identify a peer set of apps with similar functionality and compare a developer’s permission requests to that of their peers. If a very large percentage of these similar apps are not asking for a permission, and the developer is, we then let the developer know that their permission request is unusual compared to their peers. Our determination of the peer set is more involved than simply using Play Store categories. Our algorithm combines multiple signals that feed Natural Language Processing (NLP) and deep learning technology to determine this set. A full explanation of our method is outlined in our recent publication, entitled “Reducing Permissions Requests in Mobile Apps” that appeared in the Internet Measurement Conference (IMC) in October 2019.3 (Note that the threshold for surfacing the warning signal, as stated in this paper, is subject to change.)
We surface this information to developers in the Play Console and we let the developer make the final call as to whether or not the permission is truly necessary. It is possible that the developer has a feature unlike all of its peers. Once a developer removes a permission, they won’t see the warning any longer. Note that the warning is based on our computation of the set of peer apps similar to the developers. This is an evolving set, frequently recomputed, so the message may go away if there is an underlying change to the set of peers apps and their behavior. Similarly, even if a developer is not currently seeing a warning about a permission, they might in the future if the underlying peer set and its behavior changes. An example warning is depicted below.
This warning also helps to remind developers that they are not obligated to include all of the permission requests occurring within the libraries they include inside their apps. We are pleased to say that in the first year after deployment of this advice signal nearly 60% of warned apps removed permissions. Moreover, this occurred across all Play Store categories and all app popularity levels. The breadth of this developer response impacted over 55 billion app installs.3 This warning is one component of Google’s larger strategy to help protect users and help developers achieve good security and privacy practices, such as Project Strobe, our guidelines on permissions best practices, and our requirements around safe traffic handling.
Acknowledgements
Giles Hogben, Android Play Dashboard and Pre-Launch Report teams
References
[1] Modeling Users’ Mobile App Privacy Preferences: Restoring Usability in a Sea of Permission Settings, by J. Lin B. Liu, N. Sadeh and J. Hong. In Proceedings of Usenix Symposium on Privacy & Security (SOUPS) 2014.
[2] Using Personal Examples to Improve Risk Communication for Security & Privacy Decisions, by M. Harbach, M. Hettig, S. Weber, and M. Smith. In Proceedings of the SIGCHI Conference on Human Computing Factors in Computing Systems, 2014.
[3] Reducing Permission Requests in Mobile Apps, by S. T. Peddinti, I. Bilogrevic, N. Taft, M Pelikan, U. Erlingsson, P. Anthonysamy and G. Hogben. In Proceedings of ACM Internet Measurement Conference (IMC) 2019.
Facial recognition company Clearview AI hit by data theft
The startup came under scrutiny after it emerged that it had amassed 3 billion photos from social media for facial recognition software
The post Facial recognition company Clearview AI hit by data theft appeared first on WeLiveSecurity